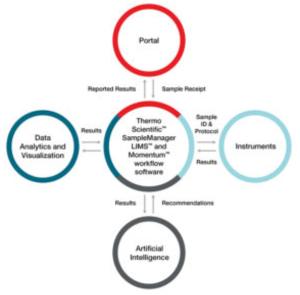
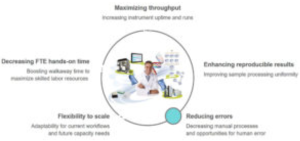
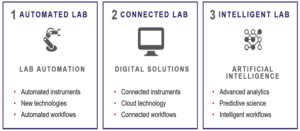
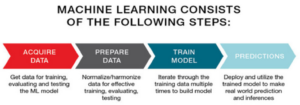
Georgian Technical University Digitalization: Accelerating The Future Of Scientific Progress Through Laboratory Automation.
Georgian Technical University Laboratory automation is driven by more than just throughput. The steps in machine learning. A holistic approach to laboratory automation builds on three foundational pillars with digitalization providing the key to unlocking the true benefits. In recent years automation has driven step changes in laboratory throughput and efficiency maximizing capacity and enabling processes that are simply not feasible using traditional manual methods. Work such as high-throughput drug discovery screening, which requires large numbers of samples and longitudinal studies involving many samples over an extended timeframe, cannot be done without automation. Although increased throughput was the initial focus for automation for today’s laboratories the need to ensure the quality, integrity and reproducibility of data – data being a laboratory’s true output – is a significant motivating force. Automation technologies are also more advanced, encompassing features such as robotics, smart workflows, advanced analytics, data visualization, natural language processing and cognitive agents. Automation is now being embraced across many different sectors, but the pharmaceutical industry has been relatively slow to adopt automated technologies. Heavy regulation may be a reason although other regulated sectors such as banking have adapted more quickly. As automation moves beyond simply enhancing individual processes its application in the life sciences can offer new and better ways to approach scientific research and development improve process reliability and consistency and shorten research timelines and iteration cycles. This discusses how integrated systems that are well defined appropriately configured and effectively implemented can drive rapid advances in scientific enterprise and it examines the crucial enabling role of digitalization technology. Why is laboratory automation important ?. While laboratory automation was originally driven by the need to increase sample and testing throughputs it offers a great deal more than simply gains in physical processing efficiency. Many tasks cannot be carried out repetitively by humans with the accuracy and speed required. Similarly minimizing human interaction reduces errors and subjectivity making results more reliable and reproducible. Intelligent automation then enables these high-quality data outputs to be tracked, managed, shared and used in multiple applications. Scientists essentially an organization’s greatest asset are freed from repetitive or basic tasks to work more efficiently and focus their skills without interruption on areas of higher value. Increasingly and especially in fields like large molecule drug discovery where small differences in structure can significantly impact efficacy and test results, process reliability and consistency are of paramount importance. Here data tracking and audit logs which connect the scientific data with the operational data are key tools in demonstrating comparability of results. Whatever the application the better data quality, integrity and reproducibility that result from automation deliver the confidence that allows faster decision-making with less need for repeat testing. These large amounts of high-quality data also feed machine learning (ML) applications and closed loop science for faster more focused scientific discovery. Furthermore laboratory automation is increasingly viewed not only as a tactical solution to address laboratory efficiency and data quality issues but also a strategic imperative in terms of business continuity, productivity and a means of gaining competitive advantage. The current pandemic has brought these issues into sharp focus and accelerated discussions on how to embrace digital transformation and automation. Georgian Technical University Laboratory automation provides extremely large amounts of high-quality data that feed a multitude of applications and help drive the speed of discovery. The ability to achieve a digital transformation and deliver this automated science is built on three foundational pillars. Georgian Technical University Physical automation the hardware that includes tools such as analytical instruments, robotic sample handling and automated reagent supply. This offers the potential to connect manually fed benchtop instruments into a connected system and drive large increases in productivity. For many people approaching automation this element will be the most familiar and easiest to understand. Georgian Technical University Data infrastructure encompassing laboratory information management systems (LIMS) to manage samples and data electronic laboratory notebooks (ELNs) dedicated connectivity tools such as Georgian Technical University Thermo Scientific Momentum workflow software and internet capable devices (internet of things IoT). Essentially the entire infrastructure that enables the generation of standardizable, sharable data and makes it available for wider use. Georgian Technical University Artificial intelligence (AI) and machine learning, deep learning technologies that take large volumes of data and turn them into the insights that drive discovery and push the science forward. Georgian Technical University true benefits of laboratory automation can be achieved only by taking a holistic approach that combines the above three elements. Within this digitalization is the crucial link. Digital science connects physical laboratory automation to the digital laboratory automation that is essential for managing and analyzing large amounts of data. Georgian Technical University Using digitalization to drive automated science. Georgian Technical University Automation projects that fail usually do so because the three pillars are not working together. For example if samples are analyzed efficiently but are not tracked effectively the ability to associate results with other data and metadata is lost and the value of all the data is diminished. In some the organic evolution of disparate digital systems and approaches can present a challenge to achieving effective digitization and the integrated operation necessary for maximum benefit. Georgian Technical University Underlying digital transformation is the concept of data key in today’s laboratory environment. Data is findable, accessible, interoperable, reusable and it is these key attributes that make it so valuable. This concept goes beyond instruments simply talking to one another. It means data must be findable, accessible between systems, scientists and be of sufficient quality to be re-used with confidence. Data management through digitalization supports the achievement of data. However many companies approaching digital transformation find that they do not necessarily have data. A recent Accenture survey found that “the majority of respondents (88% overall) note that digitalization is happening — but not broadly across the functions. Results indicate it is instead being implemented in silos that exist inside their organizations”. Data sharing platforms and enterprise-wide data strategies will be important in moving beyond this to take full advantage of all data including that which is already generated. Here integrated Georgian Technical University (exemplified by the integrated Georgian Technical University Thermo Scientific Sample software) have a key role in centralizing and managing data automating processes and delivering connectivity to provide a strong foundation for AI (Artificial Intelligence) and machine learning. Georgian Technical University Integrated centralize, manage data, automate processes and deliver the connectivity needed for AI (Artificial Intelligence) and machine learning. Georgian Technical University Successful integration of advanced digital systems with automated instrumentation not only improves data management but also process management. Connectivity of systems and data allows better instrument scheduling, resource balancing, utilization the building of feedback loops, ultimately improving efficiency and productivity. In a similar vein integrated automation provides the analytics needed to assess performance identify inefficiencies and determine the root cause of problems. Putting sensors on everything (routinely measuring laboratory temperature and humidity, for example) and feeding large amounts of diverse data into machine learning delivers previously inaccessible insights. The association of scientific data with operational data helps to fully understand processes maintain data integrity and makes root cause analysis faster and more accurate. Georgian Technical University Data integrity is a fundamental requirement in made more secure by the physical tools available today such as barcodes and tags that enable automated tracking of samples, results and the linking of them with the relevant metadata. Integrating systems, automating functions increases data integrity, ensures any errors are quickly identified and actioned. Digitalization is therefore being used to drive automated science. The IoT provides tools to generate huge volumes of research data, metadata, including operational, environmental and inventory. Processes are honed and integrity increases as biases are removed. Integrated physical and digital automation allows facilities to collaborate under standardized conditions using reliable high-quality data that can be shared and accessed across different platforms by all who need it while feeding machine learning applications. For effective laboratory automation focus on the ‘should’ not the ‘could’. Georgian Technical University While almost anything is possible with laboratory automation it is not appropriate for every process. It is therefore important to focus from the outset not on what could be done but instead on what should be done. After this initial step there are some key criteria to ensure success. The general principles are that a process should be reproducible, well-characterized, experience consistent demand and have some standardization in place. In early-stage processes immediately diving into physical automation may not be the right move simply because needs will change and even with today’s modular systems such rapid evolution presents a challenge. A degree of standardization is important. The use of standardized formats is a key requirement that is sometimes overlooked. Physical standardization (such as the use of microplates) has been critical in enabling instrument and robot manufacturers to work together to develop truly interoperable tools. Similarly standardized data formats are increasingly important to ensure high levels of data interoperability. With funding and the ability to demonstrate value cited as key barriers to digitalization there is merit in being able to reduce payback times through the implementation of standardized systems that can work across multiple disciplines. When looking to automate operations it is important to start by identifying the end goal deciding what success will look like once automation is up and running. This might be faster iteration cycles more comprehensive data higher throughput greater uptime or any number of other possibilities. Embarking upon automation without that definition is a common mistake and establishing a clear goal enables effective mapping of the right solution. Next decide who the stakeholders are and what their needs will be. Different stakeholders may have quite distinct perspectives on what they want and expect automation to achieve. The scientists needs may differ from those of the laboratory manager so on and all relevant parties and departments must be represented at the specification stage. Then identify the challenges. An upfront understanding of the manual workflow and where the challenges and potential bottlenecks lie allows decisions on whether these can be overcome with automation or if they will reduce its impact. One approach is to perform proof of concept work on individual aspects of the workflow to tackle any problems before moving ahead with full automation. This may mean conducting feasibility studies offline. The complexity of a workflow also has to be factored into the automation approach. In general a modular approach in which a complex workflow is separated into various streams provides both good automation coverage the necessary versatility avoiding the risk of building a large and inflexible monolithic system. Overall strategically driven laboratory automation with effective change management and implementation using configurable solutions that can be adapted for multiple purposes are key factors for success. From scope to implementation – choosing the right vendor. An automation vendor takes a laboratory’s goal and turns it into reality. This makes choosing the right partner for such a major undertaking a critical decision. Key considerations include the breadth of the vendor’s offering across different application areas, departments and whether they fully understand your science. Do they have the necessary solutions for cross-science application with experience in your sector and across each of the three laboratory automation pillars ? Automation solutions also need to be configurable flexible and easy to support rather than risking the creation of a monolithic bespoke system that cannot adapt to evolving needs. When it comes to implementation a vendor’s ability to provide different types of training delivered in multiple formats to match the needs of personnel at all levels and in all geographies is of the utmost importance. So too is post-installation service and support for all users. It pays to explore topics such as support channels, on-line help, community forums, response times and local resources. Increasingly important is the issue of environmental impact. With respect to the automation solution itself there are some key questions to ask: Does it allow for miniaturization and consumables re-use ? Can you separate waste streams to maximize materials recovery and ensure responsible waste treatment ? Will it support your efforts to minimize consumables and reagent usage and actively manage your effect on the environment ? As users strive to reduce their own environmental impact so the credentials of suppliers and the systems they provide must also be evaluated. Georgian Technical University Continuing the automation revolution. Many pharmaceutical companies see automation as a strategic imperative to help them remain at the cutting-edge of the industry and future-proof their laboratories. This view and has increased the urgency to adopt automation to ensure business continuity keep personnel safe and generate outputs that are beyond human capabilities. Georgian Technical University Implementing fully integrated automation solutions will inevitably entail some initial disruption particularly for larger and more complex organizations but without it discovery may be stifled. The ingredients for successful automation include a strategic drive with clear goals, senior leadership engagement and partnership with the right vendor who can offer appropriate sector experience and a range of integrated solutions that fully meet defined needs. Above all digitalization and the effective software needed to drive data is crucial to connect all elements and deliver on the ambitions promised by automated science.